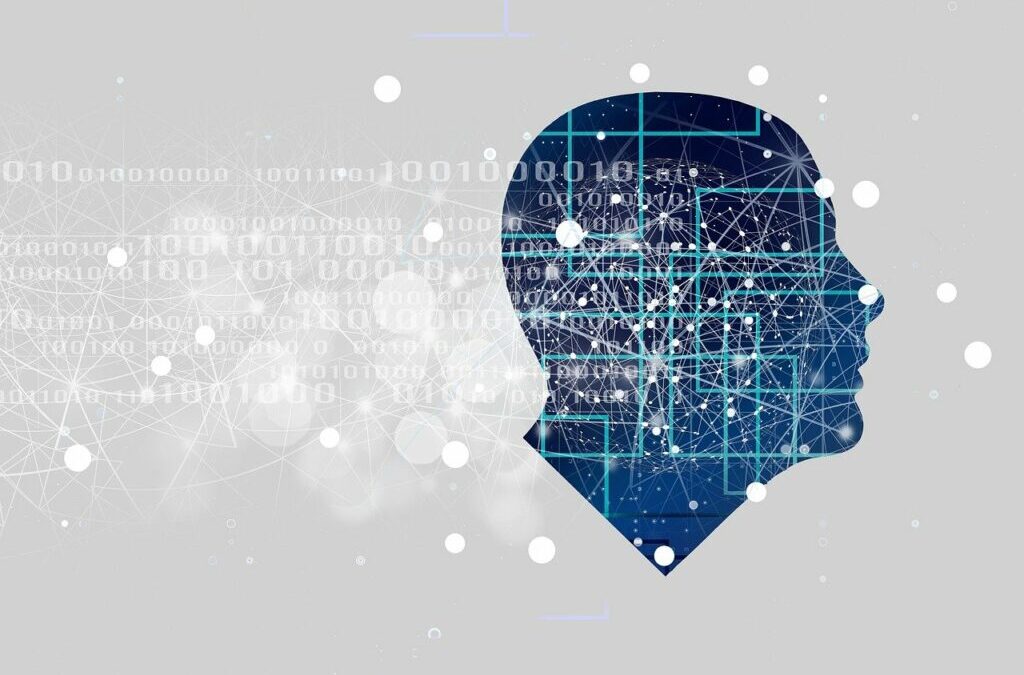
Synthetic Data
Data intelligence is crucial not only for handling COVID-19’s healthcare implications, but also for advising companies coping with emergency preparation, market continuity, product growth, and customer support during this crisis. Machine learning has the ability to change organizations privacy enhancing techniques completely, but experts in the field are still hampered by a shortage of high-quality evidence, which is the product of entirely reasonable privacy issues.
Why Synthetic Data
Synthetic data is an engineered data collection that closely resembles the original data but excludes all personal or private details that might have been contained in the raw document. To generate real – time analytics that cannot be mapped back to the original user or sale, raw data is run through special algorithms and generators.
Solutions and Opportunities
Synthetic datasets can be used successfully in several machine learning applications. If the aim of sharing a dataset is to build and test machine learning approaches for a specific task, real data is not required; a synthetic dataset that is sufficiently close to the real data will suffice. Researchers may also use simulated data to create databases that are customized to their particular needs while also being focused on real data. Various types of synthetic datasets may be developed, for example, for ICU admission forecasting, clinical trials, treatment effect estimation, and time-series data.
How do Enterprises use Synthetic Data to help them deal with the COVID recession?
Combining employment and financial data helps them to build frameworks for driving reopening preparation, such as defining companies based on their criticality and economic importance and measuring this against the degree of health risk posed to their clients. Data that is safe, available, and detailed is vital to reviving our economy. Data synthesis provides for extensive exchange of the inputs that companies and communities use to make decisions, even while reducing the risk of publicly identifiable information (PII) being mishandled or hacked by healthcare practitioners, political figures, and entrepreneurs.
Synthetic Data generation Technique Using deep learning
Variational Autoencoder (VAE) and Generative Adversarial Network (GAN) are higher credibility models that can produce synthetic results. Businesses may use a variety of approaches to complete the data synthesis process, including decision trees, big data algorithms, and iterative statistical fitting.
Conclusion
The method of generating privacy-preserving synthetic data sets is time-consuming and highly individualized. Synthesized data, on the other hand, can frequently be used for current machine learning software in a straightforward way, as well as a source of test data. This, once again, will come at the expense of privacy. Synthetic data is actually being paired with data security guarantees such as encrypted data in some technical approaches. Although the concept is admirable, there are significant time-to-market, scalability, and usability constraints. Synthetic data’s usefulness in descriptive analytics is often constrained.